Machine learning is a vibrant, fast-growing branch of artificial intelligence. It aims to make reliable decisions with real-world data, without needing to be manually programmed by a human.
The algorithms involved can be trained for specific tasks by analysing large amounts of data with some basic rules, and picking out any recurring patterns. From this analysis, they can build models that help them to identify similar patterns in new, unfamiliar data.
Whether used in voice recognition, or to identify important features in medical images — like telling skin cancer from a benign mole — machine learning is already being rolled out in many real-world applications, and its influence on our everyday lives is only set to grow in the coming years.
The challenge: In many cases, machine learning technology still isn’t ready for taking on important responsibilities. When trained, the models may pick up on different patterns than those a human would find important or relevant.
Even if a model makes a correct decision, there can be no guarantee that it came to that decision for the right reasons.
For example, a model trained to recognise tumors in MRI scans could be classifying irrelevant artefacts in the images as important, whereas a trained medical expert would know to ignore them.
Ultimately, this means that even if a model makes a correct decision – like identifying a tumor in a new scan – there can be no guarantee that it came to that decision for the right reasons.
A further problem is that machine learning models are usually trained using vast amounts of data. This makes it extremely difficult and time-consuming for users who aren’t experts in machine learning to re-evaluate their decisions manually – before deeming them trustworthy enough to be applied in real-world scenarios.
Shared Interest: In a new study released as a preprint, researchers at MIT and IBM Research, led by MIT’s Angie Boggust, present a new method for quickly and easily evaluating machine learning decisions.
Named “Shared Interest,” it is based around the concept of “saliency.” The program highlights which features in the data were important to the specific decisions that the machine learning models made.
Boggust’s team mapped out the saliency of a machine learning model tasked with identifying different vehicles or dog breeds from a series of photos.
The human researchers first made manual annotations on the images: drawing boxes around the parts of the images containing vehicles or dogs, and tagging them with the right label. This is the kind of data that is used to train machine learning models.
The program highlights which features in the data were important to the specific decisions made.
Shared Interest then compares this manual “ground-truth” data with the parts of the images that the machine learning algorithm used to make its decision – allowing Boggust’s team to quantify how well the models’ decisions aligned with theirs.
Altogether, they identified 8 recurring patterns in the models’ decisions. In one kind of error, AI models were sometimes distracted from the relevant parts of the images – entirely missing a Samoyed and an airliner — and made wrong decisions about what they contained.
In other cases, the models were correct in deciding on which parts of certain images were important — highlighting around an English setter and a chihuahua — but it still couldn’t label these breeds correctly.
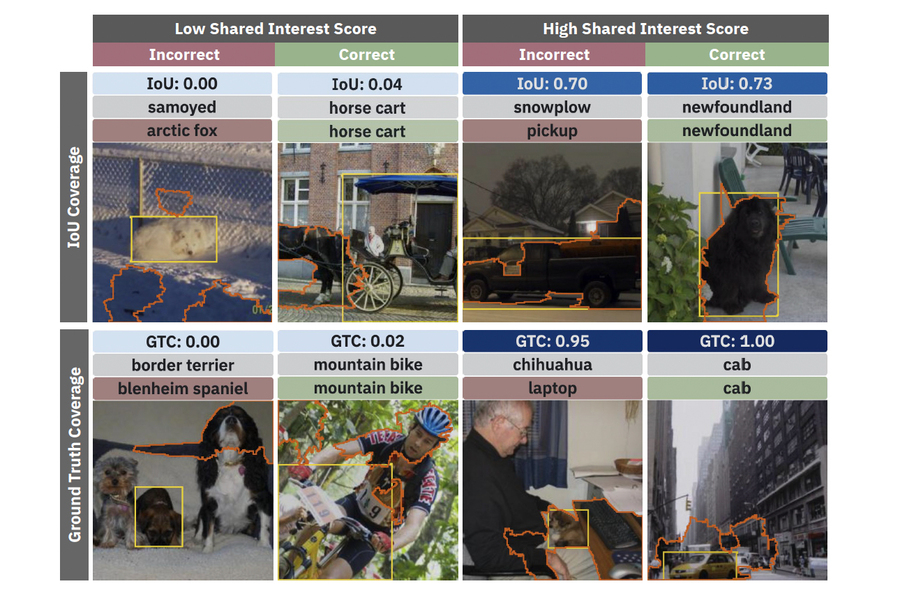
In the best case scenarios, objects, including a Maltese dog and a taxi cab, were correctly identified, based on an appropriate choice of pixels. The models and humans were looking at the same parts of the image and coming to the same conclusion.
Sometimes, however, the models correctly identified objects, including a snowmobile and a streetcar, even though the parts of the images used to make those decisions were almost completely misaligned with what humans would look for.
In other words, the model made a seemingly correct decision but for reasons that weren’t at all how humans would think about it. Those are the kinds of cases that Shared Interest is designed to red flag, rather than only focusing on whether the machine got the right outcome.
Shared Interest might one day provide assurance that machine learning models really are thinking like us.
Real-world applications: Building from these results, Boggust’s team next used Shared Interest’s in three important case studies.
In the first of these, they compared the decisions of a machine learning model with those of a medical expert, when tasked with telling the difference between benign and cancerous skin tumours from medical images. In the second, a machine learning expert used Shared Interest to analyse the saliency of a model they are developing, to assess whether it can be trusted to make decisions about real-world data. Finally, they explored a case where non-expert users could interactively annotate images – allowing them to probe how different annotations are important for triggering particular predictions.
Since Shared Interest is itself built on machine learning methods, Boggust and her colleagues acknowledge that its performance is still limited. Still, their results present promising first steps towards a robust technique for evaluating machine learning decision making.
In the future, the team hopes that Shared Interest could provide non-expert users with the assurance that machine learning models really are thinking like us, before entrusting them with high-stakes tasks.
We’d love to hear from you! If you have a comment about this article or if you have a tip for a future Freethink story, please email us at [email protected].