Every year, I join in a Yahoo! Fantasy football league, which means that every Tuesday during football season, a recap appears on my team’s page containing performance stats mixed with color commentary.
“This is one of those mistakes that you continuously think about for ten years” one of my recaps said of my decision to start Lions QB Matt Stafford over Raiders QB Derek Carr. It also noted that I “could not close out the win even with the help of DeAndre Hopkins, who scored 17.30 points.” The recap is mildly insulting even when I win, which is funny. And it contains enough statistical information that I’ve read it pretty much every week for the last two seasons. Recently, I found myself wondering who writes my recaps.
The better question, it turns out, is what writes my recaps.
Because when I scrolled down to the bottom of the page, I saw this note: “Powered by Automated Insights, the leading provider of personalized content.” So I clicked over to the company’s website, where I learned that they specialize in what’s called natural language generation. That sent me down a Wikipedia rabbit hole where I learned about computer programs that dig through data sets and then turn that information into sentences that sound like they were written by a human.
And, well, as a professional writer, I have mixed feelings about a computer doing my job. So I decided to call up Joe Procopio, Chief Innovation Officer at Automated Insights, and talk through those feelings. Our conversation, which was actually kind of inspiring, is below.
As a writer, reading about Natural Language Generation makes me very anxious, which is probably healthy. But it also got me curious. Can you talk about how Natural Language Generation works in the context of my beloved Yahoo! Fantasy recaps?
For something like Yahoo! Fantasy recaps, there’s no way actual people can write all those recaps, no matter how many writers you hired and no matter how much time you gave them. We get the previous week’s matchup data between 3 a.m. and 6 a.m. ET on Tuesday morning, and within about three hours, we’ve cranked out all the recaps we’re going to do for that day. We’re able to write about 2,000 articles per second.
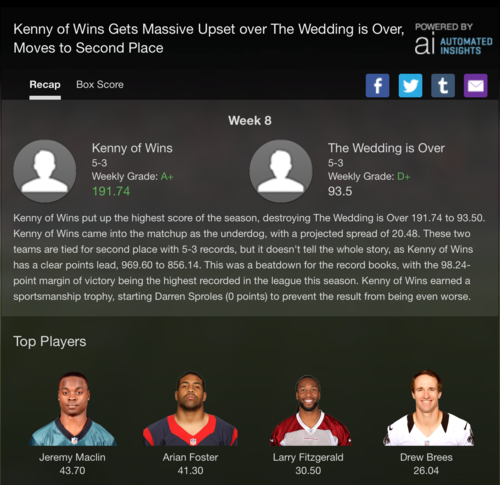
That’s where Natural Language Generation, which is the process of creating human-sounding narrative out of big data, comes in. We do best where humans can’t or won’t write.
Very early on, we realized that our best shot wasn’t recapping actual NFL games, because those games are important enough and covered enough. News organizations will send reporters or stringers. But take something like what we’re doing for the Associated Press, where we cover all the minor league baseball games. Some of those games aren’t covered by any actual humans, but all the games have data, and all the teams have fans. So we can get long-tail coverage.
To make that real-time game data more robust and more informational, we combine it with third party data. It can be geo-local or social data. It can even be weather data. And to give it more context, we try to get our hands on as much historical data as we can. For instance, our baseball data goes all the way back to the late 1800s, so we can talk about things like career milestones, franchise milestones, trends. Things that would take a long time for a human to analyze.
Over the last 12 months, our priorities have been focused on our new NLG product, which is called Wordsmith. It takes a thin slice of the the technology we use in our NLG engine and opens it up for anybody to use their own data to create their own automated content. People themselves make a huge amount of data all on their own, not to mention companies and organizations. Wordsmith takes that data and puts it in a digestible format that even a journalist can use to share with other people in a cost-effective and personalized manner.
I like this idea of mentioning journalists. It’s like co-opting the enemy.
Why do you say enemy? Because I’ve spent the last six years fighting that myth.
It’s kind of a joke. Kind of. When I saw Automated Insights wrote my fantasy recaps, it reminded me of the industry-wide anxiety that started back in 2008 and 2009, when journalists were worried about Demand Media’s content farms. They were churning out a ton of low-grade content really quickly at a super low cost. So that’s what I thought of first. But I don’t actually think NLG is the same thing. While I love good data journalism, the idea of sifting through Excel sheets is not personally exciting to me. I don’t mind letting Automated Insights do that work.
We see that a lot. Think of the Associated Press. When Lou Ferrara brought us on to do the AP’s quarterly finance reports, he had no qualms about letting people know that this was a computer-generated article produced by Automated Insights. In fact, he gave Automated Insights credit on each of those articles.
Before Automated Insights, the AP was able to cover around 400 quarterly earning press releases…With us, they’re able to cover 4,500-plus.
Before Automated Insights, every quarter, the AP was able to cover around 400 quarterly earning press releases, each quarter. With us, they’re able to cover 4,500-plus, and that’s only limited by what they actually want to cover. That freed Lou’s journalists up to do actual journalism: To look at the how and the why, while we presented the facts, looked for trends, milestones, changes, and what that meant for the price of stocks. We do all that math so that within six seconds of the data being released, we were able to produce a 500-to-1000-word-recap of that quarterly release. And then the AP could either pass that along to their distribution partners, or hold it and get quotes. Or their distribution partners could then get quotes. They could do the art and science of journalism, while we took the math out of it. He didn’t have to let anybody go, and his team got to be more productive.
We also do very well in business intelligence, where we’re not really threatening anyone’s job, except maybe the data scientist’s, except they tend to appreciate us, too, because it allows them to take time to hypothesize, theorize, and draw conclusions.
One of the things I notice when I read anxious writing about automation and robotics is zero-sum thinking. But people who love to write, or don’t think they know how to do anything else, seem to forget that they’re information consumers, too. Before I can share anything with my readers, I have to first consume it and understand it. NLG seems like it could inform writing rather than replace it.
I don’t think there are too many people for whom writing is the only skill they have. Even in journalism, writing is just a part of that overall skillset. It’s not the only thing they do. Maybe if you’re writing fiction, it’s the only thing you do, but even then, you’re doing creative things that make you better than someone else. But writing as a mechanical exercise hasn’t changed for a very long time. And the things that are threatening sports journalism, for instance, have to do with plummeting ad sales. The changes in sports broadcast journalism–the Hollywoodization of that field–has nothing to do with automation.
Horse-and-buggy manufacturers lost their minds when Henry Ford came out with the Model T. The question then becomes: Do you want to build a faster horse-and-buggy, or do you want to start learning about how these cars work?
That said, I do understand the automation principle. It’s coming to white collar and thinking careers the same way it did for manufacturing and blue collar careers in the 70s and 80s. That’s what happens. Horse-and-buggy manufacturers lost their minds when Henry Ford came out with the Model T. The question then becomes: Do you want to build a faster horse-and-buggy, or do you want to start learning about how these cars work?
I think more people are jumping into that. We all have computers in our pockets now. It’s not as scary as was 10 years ago.
It’s funny that you bring up poetry and fiction. If a writer is at the top of the writing skill hierarchy, they’re maybe not too anxious about the bottom getting eaten away by automation. But then I wonder: Could NLG tell good fiction stories?
Perhaps. But poets and fiction writers can take relief in the fact that machines are terrible at that kind of creativity, and I don’t think I’ll live long enough to regret saying that they’ll never be good at it.
To me, there’s a human element that goes into the artistic side of writing that machines can’t replicate. Once computers understand the human condition, then I think we’ve got a better shot at it. But we’re not at artificial intelligence yet, we’re at machine learning, which is derivative of AI. Computers don’t think, and I’m not sure they ever will the way a human thinks. And I also write. I write fiction, I write columns, and I don’t feel threatened by this technology.
When you think of…the art of writing, you’re talking about endless possibilities for creativity, which machines are terrible at.
I think what machines are always going to be really good at is providing information about large sets of data. And as machines evolve, the larger those data sets are, the more we’ll be able to get from them. But if you look at machine learning, you see machines are good at doing one niche thing really well. If you give them more than one task, they start to falter. When you think of writing–not just the mechanics of constructing a sentence–but the art of writing, you’re talking about endless possibilities for creativity, which machines are terrible at. Granted, we’ve taught our machines to be creative, but there’s a limit too. A machine isn’t making decisions about how snarky to be when it sends you your draft recap. Humans are telling it how snarky to be based on the data.
There seems to be a tonal difference between what I might get using Wordsmith, which is Automated Insight’s plug-and-play content creator, and what gets generated by Automated Insight’s NLG engine for Yahoo! What explains that difference?
We wanted to make something the average person could use to do automated content without spending six or seven figures.
When we do something like a fantasy football recap, that’s our writers coding in NLG. Wordsmith is a more template-based approach. We did that because a template-based approach is much easier to understand. It doesn’t take much to understand branching, but in terms of the code we use in the NLG engine, that takes understanding machine learning concepts and programming concepts, which eliminates a good portion of the population. We wanted to make something the average person could use to do automated content without spending six or seven figures. Like, maybe there’s an automated report they have to do 50 times a month, or product descriptions or stock reports. A template-based approach made more sense.
We want to make Wordsmith work more and more like our Natural Language Generation engine without having that be a burden on the user. So we’re using machine learning to make Wordsmith suggestive and predictive about what the user is trying to communicate. Eventually, our bet is that Wordsmith users will have the same kind of content-creation power that we have when we make content using our NLG engine.
Where is NLG not being used that it could be or should be used?
One of the interesting developments about NLG over the last three years is that it really is no longer vertical specific. We were called Statsheet when we started, and did college basketball statistics. When I got involved six years ago, we started doing automated content. Then after our first round of fundraising, we changed our name to Automated Insights and went vertical agnostic. So we’re seeing opportunities for NLG in more places, but we’re still only scratching the surface.
When people download data with Wordsmith, we ask them to tell us what industries they work in. And we got 46 different answers. Forty-six different industries have found a use for NLG. Finance, insurance, banking, business intelligence, personal fitness, sports. It really runs the gamut of things you might not expect to be handled by NLG. As we’re getting so much more data, we’re finding uses everywhere.
I’m a personal fitness junkie. Tell me about the opportunities there.
If you use RunKeeper, you’re generating lines and lines and lines of data as you run. Everything from your pace, elevation, heartbeat, location, all of this is being recorded while you run. You’ve now got thousands of lines of data from a 30 minute run, and there’s so much you can do with that, especially when you compare it to previous runs, your age, your demographic, your personal best. If we go out to third party data, we can generate reports about how much tread is on your shoes. It’s limitless possibilities.

Telling me what all that means is not anyone’s job right now, so it’s not really displacing a person.
That’s right. And I’m kind of shocked that no fitness tracker maker has an NLG solution built into the app. Because when I’m done that’s the first thing I do — run my workout through Wordsmith and get my report. And Wordsmith users can do that. They can get a report emailed to them. Right now, you couldn’t have that information without a personal trainer or a data scientist who could crunch numbers and analyze performance and get back to you. That’s the kind of thing we’re making more cost effective. And I don’t think in either case we’re threatening their jobs.
Do you think there’s a tension, or maybe a need for balance, between visual representations of data and written interpretations of data? I ask because we seem to be in an age of charts. And sometimes that comes at the expense of narrative analysis.
We’ve created reports for marketing companies…and it’s saved them literally hundreds of hours a month of report writing.
It’s very important. Google Analytics is a great example. It gives you a ton of visual information and a ton of data, but not a lot of narrative explanation of what you’re seeing. We’ve created reports for marketing companies that then turn those around to clients, and it’s saved them literally hundreds of hours a month of report writing. That was a stepping stone to letting everyone write their own reports. That’s where the science is headed.
As data gets more and more complex, you have to have visual representations of visual representations. If you look at R and plot graphs, with people able to break down their analysis to such a local level, that’s where you need written language to give you context around those charts. The color scale off to the right doesn’t really interpret it with much value at higher levels of complexity.
Thinking about the complexity of the Google Analytics dashboard makes me wonder what the opportunities are for using NLG to answer questions I didn’t think to ask.
We love outliers and edge cases. Those are where cool things happen in the world of data science. I take everything back to sports, but when a quarterback has a seven-touchdown game, that’s an outlier. We plan for outlier moments, and thanks to machine learning, our system is now looking for those kinds of things. We, the people, don’t have to be predictive going in. If the machine learning is right, it’ll recognize that something unexpected has happened. And it can, within a certain degree of accuracy, suggest or even predict what it means or what caused it. That’s not even NLG, that’s data science. But once we can make those kinds of algorithmic predictions, NLG can then parse that for the reader. Instead of just color-coding or starring a phenomenon, NLG can tell you, “Here’s what happened, here’s the last time it happened, and here’s why it appears it may have happened.”
I’d love to see this applied to NBA basketball. One of the first things I do when a player gets six or seven steals in a game is go look up the last time something like that happened.
Since being acquired by a private equity firm, we’ve started working with STATS, and one of the things we’re really pumped about is what they’re doing with SportVU, which is the six cameras on a basketball court that track all the players as well as the movement of the ball. That system produces a million rows of data per game. And it can range from how much a player is slowing down due to an ankle injury, can they make that drive to the basket that they made before. How many offensive rebounds were contested? How many blocked shots result in a turnover instead of going out of bounds? So you’re starting to get really true statistics related to performance because of this elite data collection.
We can do a lot of other fun stuff. We play a lot of ping-pong here, and we did this hackathon where we took a camera that’s used professionally to detect a fault, and we hooked it up to Wordsmith. We hooked all that up to Alexa, which is Amazon’s voice emanation device. And then we used Periscope to livestream a ping-pong game with color commentary provided by Alexa, written by Wordsmith, based on data from this camera. This is just two of our employees in the breakroom, and Wordsmith is writing statistics like, “When Ganesh gets a point on his first serve, he gets a point on his second serve 60% of the time.”